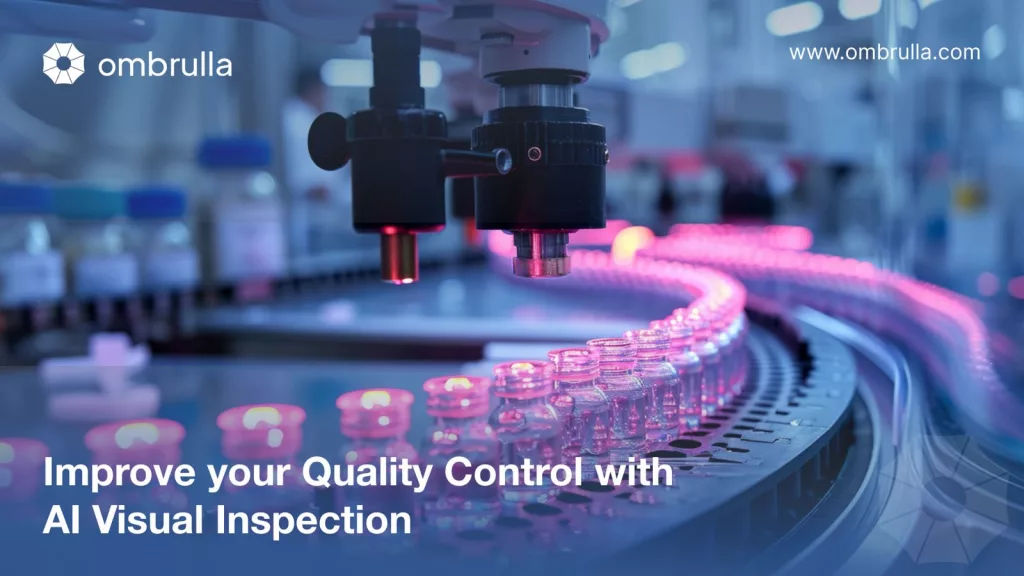
In today’s manufacturing industry, traditional quality control methods relying on manual inspection face numerous challenges, such as inefficiency, human error, and inconsistent results. These problems often lead to undetected defects, wasted resources, and disruptions in production. However, with the advent of artificial intelligence, these issues are being addressed more effectively than ever before.
AI-based visual inspection revolutionizes quality control by integrating advanced computer vision and machine learning algorithms to detect defects swiftly and accurately. This automated visual inspection minimizes errors and optimizes resource utilization, ensuring continual improvement in product quality over time.
Artificial intelligence’s speed and precision replace the inefficiencies of manual inspection, fundamentally transforming quality inspection processes. Real-time analysis enables manufacturers to swiftly identify anomalies, reducing production disruptions seamlessly. Additionally, iterative learning empowers organizations to refine inspection protocols, sustaining excellence in product quality effectively.
Embracing AI Visual Inspection in manufacturing for quality inspection isn’t merely an evolution but a revolution in achieving manufacturing excellence. The seamless integration of AI vision systems streamlines operations, mitigates risks, and enhances competitiveness in today’s dynamic market landscape. This technological advancement represents a significant step forward in maintaining comprehensive product quality standards through cutting-edge computer vision applications.
Table of Contents
Understanding AI Visual Inspection
AI visual inspection utilizes computer vision, machine learning, and deep learning algorithms to analyze visual data. These technologies enable machine vision systems to make decisions based on what they “see.” Computer vision allows machines to interpret and understand images or videos. Machine learning algorithms enable them to learn from data and improve performance over time.
Deep learning algorithms, a subset of machine learning, further enhance the AI’s ability to extract meaningful insights from visual data. By employing computer vision, machine vision systems can detect defects or anomalies in products with precision. Machine learning algorithms recognize patterns and variations in visual data. This allows for accurate identification of quality issues.
Deep learning algorithms mimic the way the human brain processes information. They enhance AI’s ability to make nuanced decisions based on complex visual data. AI visual inspection revolutionizes quality control processes by automating the analysis of visual data. Through integrating computer vision, machine learning, and deep learning algorithms, computer vision systems efficiently identify defects.
This technology enhances accuracy and improves the speed and efficiency of quality control operations. Ultimately, this leads to higher product quality and increased customer satisfaction. AI in manufacturing for quality inspection isn’t just an evolution. It is a revolution that significantly impacts product standards and market competitiveness.
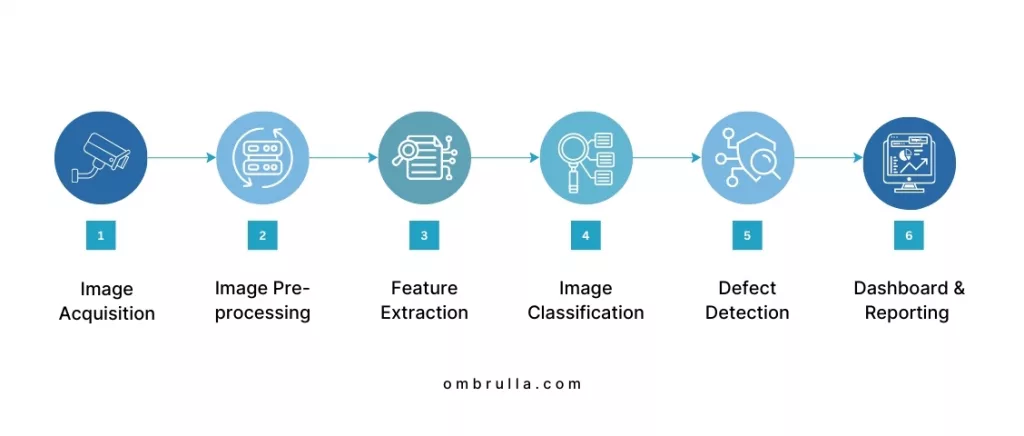
The step-by-step process of AI visual inspection involves several key stages. First, image acquisition captures high-quality images or videos of the products. Next, preprocessing enhances the images for better analysis. Then, feature extraction identifies key characteristics of the images.
After feature extraction, the AI system classifies the images based on trained models. This helps in identifying defects and anomalies. Finally, the system generates a report detailing the inspection results. This report informs the quality control team of any issues found during the inspection.
In essence, Artificial Intelligence inspection revolutionizes quality control processes by automating the analysis of visual data. Through the integration of computer vision, machine learning, and deep learning algorithms, computer vision systems can efficiently identify defects and anomalies in products. This technology not only enhances accuracy but also improves the speed and efficiency of quality control operations, ultimately leading to higher product quality and increased customer satisfaction.
Step-by-step process of AI Visual Inspection
1. Image Acquisition: High-quality images or videos of products are captured using cameras. The setup includes lighting to ensure clear and consistent image quality. Proper positioning of the cameras is crucial for capturing all relevant angles. This step ensures that the AI visual inspection system has accurate data to analyze.
2. Preprocessing: Images are enhanced to improve clarity and highlight relevant features. Techniques like noise reduction and contrast adjustment are used. This step ensures that any distortions or irrelevant information are minimized. Enhanced images make it easier for the AI system to analyze the data accurately.
3. Feature Extraction: Key characteristics of the images are identified for further analysis. Algorithms detect specific patterns, shapes, and textures in the images. This step transforms raw image data into a format suitable for machine learning. Feature extraction is critical for identifying significant details in the visual data.
4. Classification: The AI Defect Detection system classifies images based on trained models to identify defects. The system compares features from the images with known patterns of defects. This step helps in sorting products into categories such as defective or non-defective. Accurate classification is essential for effective quality control.
5. Defect Detection: The system detects any anomalies or defects in the products. Advanced algorithms analyze the extracted features to spot irregularities. This step involves comparing current images to a database of defect patterns. Quick and precise defect detection helps in maintaining high product quality.
6. Reporting: A detailed report of the inspection results is generated for the quality control team. The report includes information on detected defects and their locations. This step provides actionable insights for quality improvement. Timely reports help in addressing issues promptly and maintaining production standards.
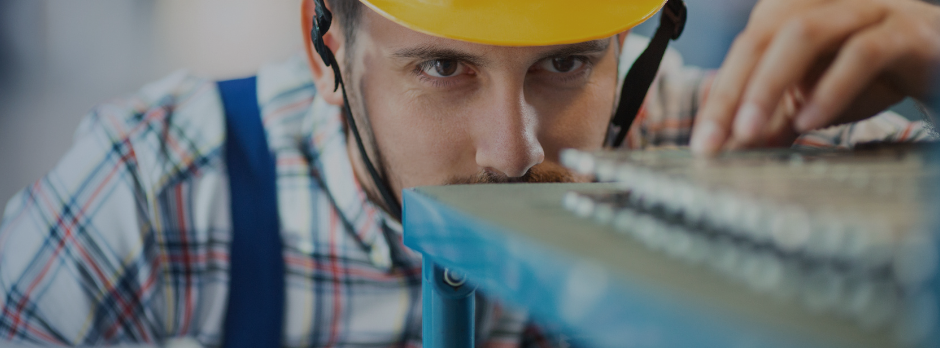
Enhance your Quality Control Using Artificial Intelligence
Benefits of AI Visual Inspection in Quality Control Process
AI visual inspection offers numerous benefits for quality control processes, including enhanced speed, accuracy, consistency, and scalability. Businesses implementing AI visual inspection systems have reported significant improvements in their quality control operations. For instance:
1. Increased Accuracy: AI visual inspection significantly improves the accuracy of defect detection. It reduces human error by consistently analyzing visual data with precision. This leads to a higher identification rate of defects. Increased accuracy ensures that only quality products reach the market.
2. Enhanced Speed: AI systems analyze images much faster than manual inspections. Real-time processing allows for immediate detection of anomalies. This speed helps in maintaining a smooth production flow. Enhanced speed in inspections reduces delays and increases overall efficiency.
3. Consistency: AI visual inspection provides consistent results, regardless of external factors. Unlike human inspectors, AI systems do not suffer from fatigue or distractions. This consistency ensures uniform quality control across all products. Reliable inspection processes maintain high standards.
4. Cost Efficiency: Implementing AI visual inspection can reduce operational costs. It decreases the need for extensive human labor in inspection tasks. Over time, this leads to significant savings in labor costs and error-related expenses. Cost efficiency makes AI visual inspection a valuable investment for manufacturers.
5. Scalability: AI systems can easily scale to inspect large volumes of products. They handle increased production demands without compromising inspection quality. This scalability is crucial for manufacturers looking to expand operations. It ensures that quality control keeps pace with production growth.
6. Data Collection and Analysis: AI visual inspection systems collect and analyze large amounts of visual data. This data provides insights into defect patterns and trends. Manufacturers can use these insights to improve production processes and reduce defects. Data-driven decisions enhance overall product quality.
7. Continuous Improvement: AI systems learn and improve over time through machine learning. They adapt to new defect types and refine their detection algorithms. This continuous improvement ensures that the inspection process evolves with changing production needs. Ongoing enhancements maintain high quality and adapt to new challenges.
Case Studies and Success Stories
Case Study 1: Steel Rail Inspection in Steel Industry
In the steel industry, implementing AI visual inspection for steel rail inspection has yielded remarkable results. By employing AI-driven inspection solutions, steel manufacturers can swiftly detect defects and anomalies in steel rails with unparalleled precision. This technology has led to a significant reduction in manual inspection time and improved overall product quality.
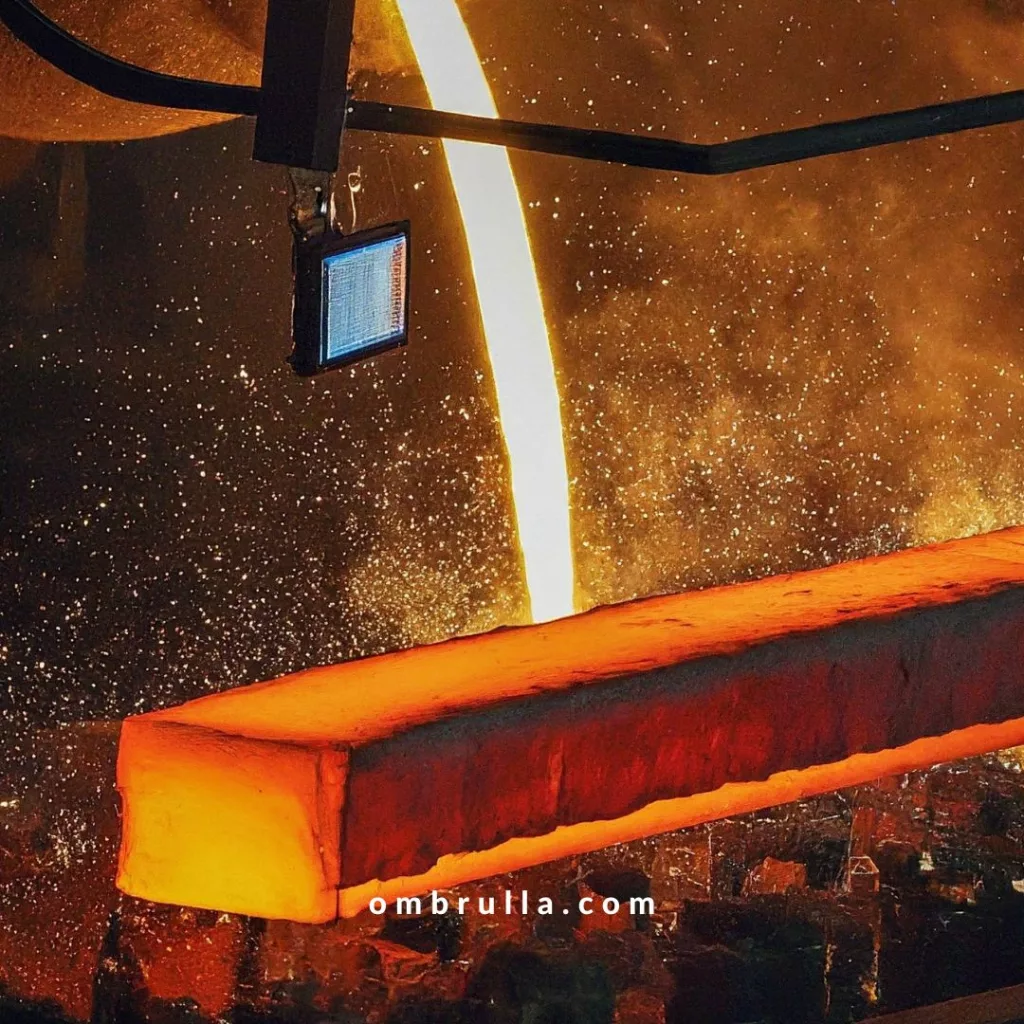
Moreover, the integration of AI visual inspection has resulted in substantial cost savings for steel manufacturers. With fewer defects escaping detection and timely identification of quality issues, the need for costly rework and repair has diminished considerably. As a result, companies have reported increased operational efficiency and higher customer satisfaction levels.
Problem Statements in Steel Rail Inspection
1. Inconsistent Quality Control:
Manual inspections varied due to human factors such as fatigue and subjectivity. These inconsistencies often led to undetected defects, compromising the quality of steel rails.
2. High Labor Costs:
Manual inspections required significant human resources. The high labor costs associated with these inspections impacted overall profitability.
3. Slow Inspection Processes:
Traditional inspection methods were time-consuming, slowing down the production process. Delays in inspection caused bottlenecks in the manufacturing line, affecting overall efficiency.
4. Missed Defects:
Subtle defects often went unnoticed during manual inspections. Missed defects led to higher rates of defective products, resulting in customer complaints and returns.
5. Inability to Scale:
Increasing production demands overwhelmed the manual inspection capabilities. The system struggled to maintain quality standards at higher production volumes.
Implementation of AI Visual Inspection
Step 1: Feasibility Study and Planning
A thorough feasibility study was conducted to understand the specific requirements for steel rail inspection. Critical points in the production process where AI inspection would be most beneficial were identified.
Step 2: System Integration
High-resolution cameras were integrated at key stages of the production line, particularly in areas where surface and structural integrity of the rails are checked. Proper lighting and camera positioning were ensured to capture clear images of the steel rails.
Step 3: Preprocessing and Data Preparation
Preprocessing techniques, such as noise reduction and contrast adjustment, were implemented to enhance image quality. Algorithms were developed to handle variations in lighting and surface conditions, ensuring accurate analysis.
Step 4: Feature Extraction and Model Training
Key features from images, such as surface texture, edge profiles, and dimensional measurements, were extracted. Machine learning models were trained using a comprehensive dataset of known defect patterns and dimensions.
Step 5: Real-Time Inspection and Classification
AI models were deployed to classify images in real-time, identifying surface defects, dimensional inaccuracies, and internal flaws. The system flagged defective products for further inspection or rework, ensuring only high-quality rails proceeded.
Step 6: Continuous Monitoring and Improvement
The system’s performance was continuously monitored, and data was collected for ongoing improvement. Machine learning was used to refine detection algorithms and adapt to new defect types over time.
Results and Benefits
Increased Accuracy:
The AI system significantly improved the detection rate of surface and internal defects in steel rails. Consistent inspections reduced the number of defective products reaching customers.
Enhanced Speed:
Real-time analysis sped up the inspection process, reducing delays and bottlenecks. This led to increased throughput without compromising quality control.
Cost Efficiency:
Reduced reliance on manual labor decreased operational costs. Savings on labor and reduced waste improved overall profitability.
Scalability:
The system easily scaled with increasing production demands. It maintained high quality standards even at higher volumes, ensuring the inspection process kept pace with production growth.
Data-Driven Insights:
Collected data provided insights into defect patterns and production issues. This enabled proactive measures to prevent recurring defects and improve processes, leading to overall enhancements in product quality.
Customer Satisfaction:
Higher quality products led to increased customer satisfaction. Reduced complaints and returns strengthened the brand’s reputation, fostering greater trust with customers.
Case Study 2: Fabric Inspection in Textile Industry
In the textile industry, AI visual inspection has revolutionized fabric inspection processes. By leveraging AI-driven solutions, textile manufacturers can meticulously examine fabrics for defects, such as tears, stains, or irregularities in patterns, with exceptional accuracy. This has enabled them to maintain stringent quality standards and ensure that only flawless products reach the market.
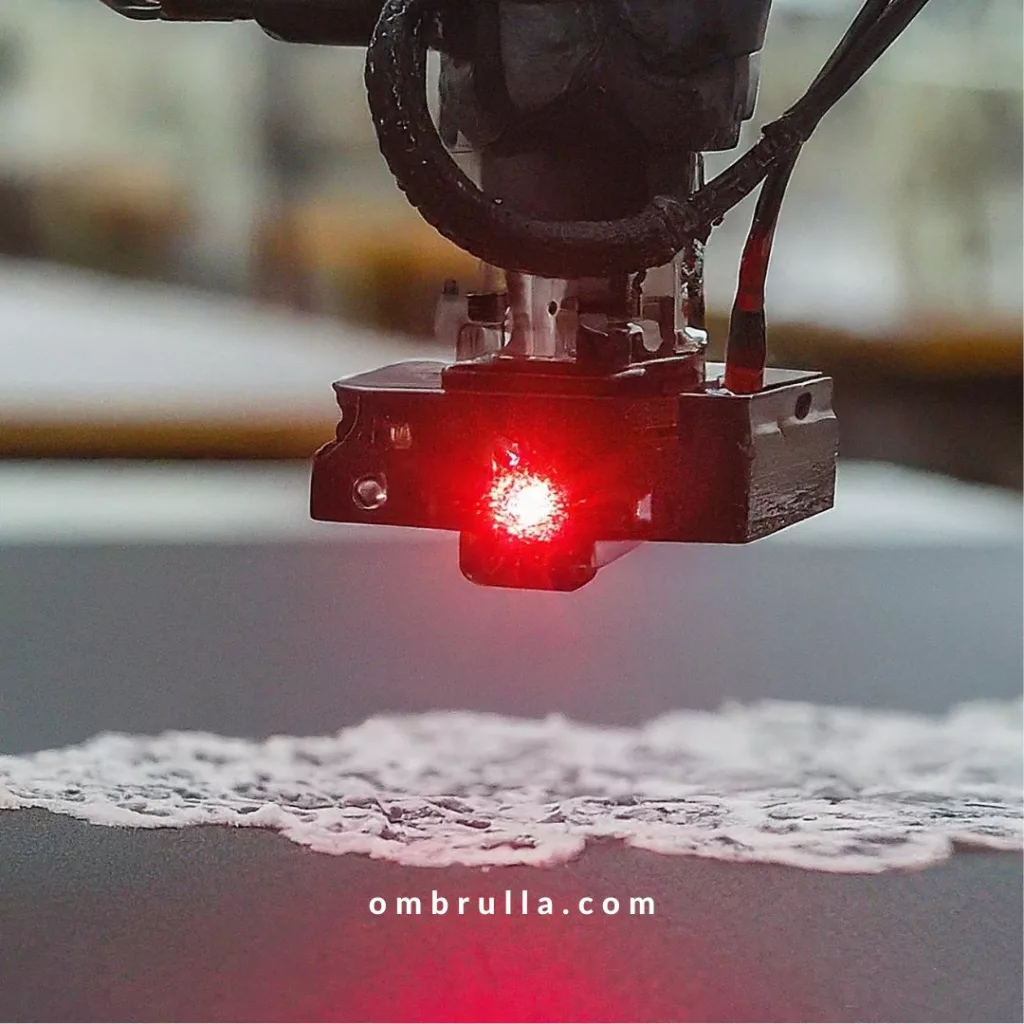
Furthermore, the adoption of AI visual inspection has streamlined production workflows and enhanced efficiency in the textile industry. With automated inspection processes, manufacturers can handle larger volumes of fabric without compromising on quality. This has resulted in increased productivity and reduced lead times, ultimately driving profitability and competitiveness in the market.
Problem Statements in Fabric Inspection
Variability in Manual Inspections:
Manual inspections vary due to human factors such as fatigue and subjectivity. These inconsistencies can lead to undetected fabric defects, affecting the overall quality of textile products.
Significant Human Resource Expenses:
Manual fabric inspections require significant human resources. High labor costs impact operational expenses and reduce profitability for textile manufacturers.
Time-Consuming Inspection Methods:
Traditional methods of fabric inspection are time-consuming, which slows down production. Delays in inspection processes can lead to bottlenecks in manufacturing lines and impact overall efficiency.
Undetected Fabric Imperfections:
Subtle defects in fabric texture, color, or weave pattern may go unnoticed during manual inspections. These defects can result in higher rates of defective products and customer dissatisfaction.
Challenges in Production Volume Management:
As production volumes increase, manual inspection processes struggle to maintain consistent quality control standards. This scalability issue hinders production efficiency and may compromise product quality.
Implementation of AI Visual Inspection
Step 1: Feasibility Study and Planning
Conducted a comprehensive feasibility study to assess the specific requirements for fabric inspection in textile manufacturing. Identified critical inspection points and types of defects that AI visual inspection systems could effectively detect.
Step 2: System Integration
Integrated high-resolution cameras and sensors at strategic points along the fabric inspection line. Ensured optimal lighting and camera positioning to capture clear and detailed images of fabric surfaces.
Step 3: Preprocessing and Data Preparation
Implemented preprocessing techniques to enhance image quality and consistency. Algorithms were developed to normalize lighting conditions, remove noise, and adjust contrast for accurate analysis.
Step 4: Feature Extraction and Model Training
Extracted key features from fabric images, such as weave patterns, thread density, and color uniformity. Trained machine learning models using a diverse dataset of fabric defects and quality standards.
Step 5: Real-Time Inspection and Classification
Deployed AI models to analyze fabric images in real-time, identifying defects such as stains, tears, uneven dyeing, or weaving irregularities. Automated classification and tagging of defective fabric rolls for further inspection or rework.
Step 6: Continuous Monitoring and Improvement
Continuously monitored the performance of AI visual inspection systems. Collected data on detected defects and inspection outcomes to refine algorithms and improve detection accuracy over time.
Results and Benefits
Increased Accuracy:
The AI visual inspection system significantly improved the detection accuracy of fabric defects, reducing the occurrence of defective products reaching consumers.
Enhanced Efficiency:
Real-time analysis accelerated the fabric inspection process, reducing production delays and enhancing overall manufacturing efficiency.
Cost Savings:
Reduced dependency on manual labor lowered operational costs associated with fabric inspection. Improved resource utilization and minimized waste improved profitability.
Scalability:
The AI system easily scaled to accommodate increasing production demands without compromising inspection quality or speed.
Data-Driven Insights:
Generated valuable insights into fabric defect patterns and production issues. Enabled proactive measures to prevent defects and optimize manufacturing processes.
Customer Satisfaction:
Higher quality fabrics contributed to increased customer satisfaction and loyalty. Reduced defects and improved consistency bolstered brand reputation and market competitiveness.
Case Study 3: Automobile Paint Inspection Using AI Visual Inspection
In the automotive industry, AI visual inspection has played a pivotal role in improving paint quality control processes. By deploying AI-driven inspection systems, automotive manufacturers can identify imperfections in paint finishes, such as scratches, uneven coating, or color discrepancies, with exceptional precision. This has enabled them to deliver vehicles with flawless paint finishes, meeting customer expectations and enhancing brand reputation.
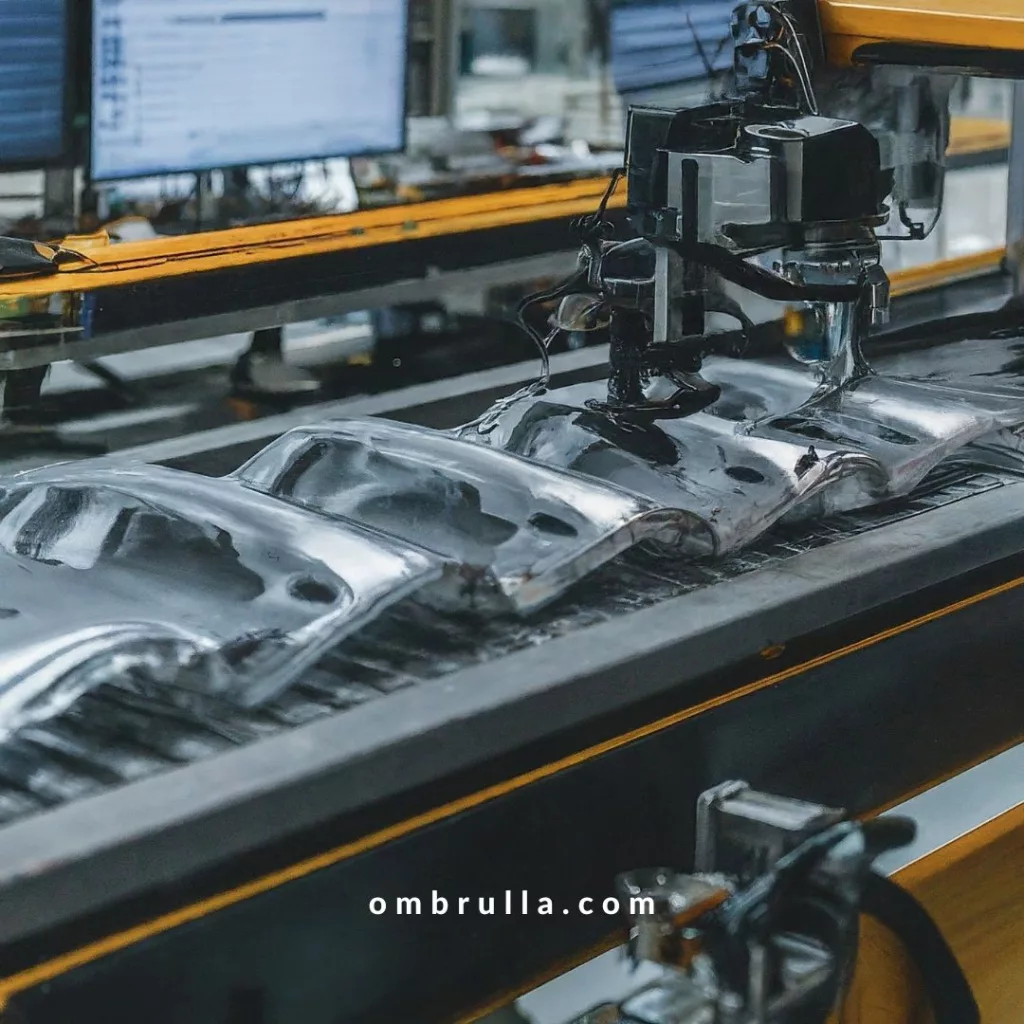
Moreover, the integration of AI visual inspection has led to significant cost savings for automotive manufacturers. By reducing the occurrence of paint defects and minimizing rework requirements, companies have realized substantial savings in both material costs and labor expenses. Additionally, the implementation of AI-driven inspection solutions has contributed to faster production cycles and improved throughput rates, enabling manufacturers to meet market demand more efficiently.
Challenges in Automobile Paint Inspection
Quality Consistency:
Manual inspections suffer from inconsistencies due to human factors such as fatigue and subjectivity. These variations can result in overlooked defects or uneven paint quality across vehicles.
Labor Intensiveness:
Manual paint inspections require a substantial workforce, contributing to high labor costs. This impacts overall profitability and increases the expense of maintaining quality control measures.
Slow Inspection Processes:
Traditional inspection methods are time-consuming, causing production line delays. Slower inspections can lead to bottlenecks and reduced manufacturing efficiency.
Defect Detection Accuracy:
Subtle paint defects, including scratches, bubbles, or inconsistent coatings, may be missed during manual inspections. These defects can compromise the visual appeal and durability of vehicle paint finishes.
Scalability Challenges:
Scaling up production often overwhelms manual inspection capabilities, resulting in compromised quality control and inconsistent inspection outcomes.
Implementation of AI Visual Inspection
Feasibility Assessment and Planning
Conducted a thorough feasibility study to evaluate the suitability of AI visual inspection for automobile paint inspection. Identified critical stages in the paint process where AI could effectively detect and classify defects.
System Integration and Setup
Integrated high-resolution cameras and optimized lighting setups at strategic locations within the paint booth and assembly line. Ensured precise camera positioning to capture detailed images of vehicle paint surfaces.
Image Preprocessing and Data Preparation
Implemented advanced preprocessing techniques to enhance image clarity, reduce noise, and standardize lighting conditions. Developed algorithms to mitigate reflections and other surface distortions.
Feature Extraction and Model Training
Extracted relevant features from paint images, such as color consistency, texture, and surface finish. Trained machine learning models using a comprehensive dataset of paint defect examples to enhance detection accuracy.
Real-Time Inspection and Classification
Deployed AI models for real-time analysis of paint images, enabling automated defect classification. The system promptly identifies and flags vehicles requiring further inspection or corrective action.
Continuous Monitoring and Improvement
Continuously monitored system performance and gathered data to refine detection algorithms and adapt to emerging defect patterns. Leveraged machine learning for ongoing optimization and enhanced inspection capabilities.
Results and Benefits
Improved Defect Detection Accuracy:
AI visual inspection significantly enhanced the identification of paint defects compared to manual methods. This resulted in fewer defective vehicles reaching consumers, thereby improving overall product quality.
Enhanced Operational Efficiency:
Real-time analysis accelerated paint inspection processes, minimizing production delays and enhancing manufacturing throughput. This efficiency boost contributed to overall cost savings and improved profitability.
Cost Efficiency:
Reduced reliance on manual labor decreased labor costs associated with paint inspection. Lower rework expenses and improved resource allocation further contributed to enhanced cost efficiency.
Scalability and Consistency:
The AI system seamlessly scaled with increasing production demands, maintaining consistent paint quality across higher volumes of vehicles. This ensured that stringent quality standards were upheld during periods of production growth.
Actionable Insights and Proactive Maintenance:
Generated valuable data insights into paint defect trends and production inefficiencies. This enabled proactive measures to prevent recurring defects and optimize paint application processes, fostering continuous improvement in paint quality and operational excellence.
Customer Satisfaction and Brand Reputation:
Higher-quality paint finishes contributed to increased customer satisfaction and loyalty. Reduced instances of paint defects minimized customer complaints and bolstered brand reputation in the competitive automotive market.
Conclusion
In conclusion, integrating AI visual inspection into your quality control process is essential for staying competitive in today’s manufacturing landscape. By leveraging advanced technologies like computer vision and machine learning, businesses can enhance efficiency and accuracy while reducing manual labor and costs associated with traditional inspection methods.
Furthermore, AI visual inspection enables manufacturers to maintain consistent quality standards and meet customer expectations. With rapid detection of defects and anomalies, companies can mitigate risks of product recalls and ensure brand reputation remains intact. Embracing this technology is not just a means of improvement, but a strategic imperative for long-term success in the industry.
Ultimately, the adoption of AI quality inspection represents a significant step towards modernizing quality control practices. As manufacturing processes continue to evolve, embracing innovative solutions becomes imperative for sustaining competitiveness. By integrating AI visual inspection, businesses can unlock new levels of efficiency, accuracy, and profitability, positioning themselves as leaders in the ever-changing manufacturing landscape.
AI Applications AI Data Analytics AI Defect Detection AI forecasting AI for Everyone AI in Automotive AI in Green Logistics AI in Logistics AI in Manufacturing AI in Pharmaseutical AI Inspection AI in Supply chain AI Quality Control AI Quality Controll AI Quality Inspection AI Video Analytics AI Visual Inspection AI Visual Inspection use cases Artificial Intelligence asset health monitoring asset performance management asset performance management software asset tracking Computer Vision Defect Detection equipment tracking Future of AI Google Industrial AI iot asset management Predictive maintenance predictive maintenance systems Process Optimization Ship Inspection using AI
Leave a Reply