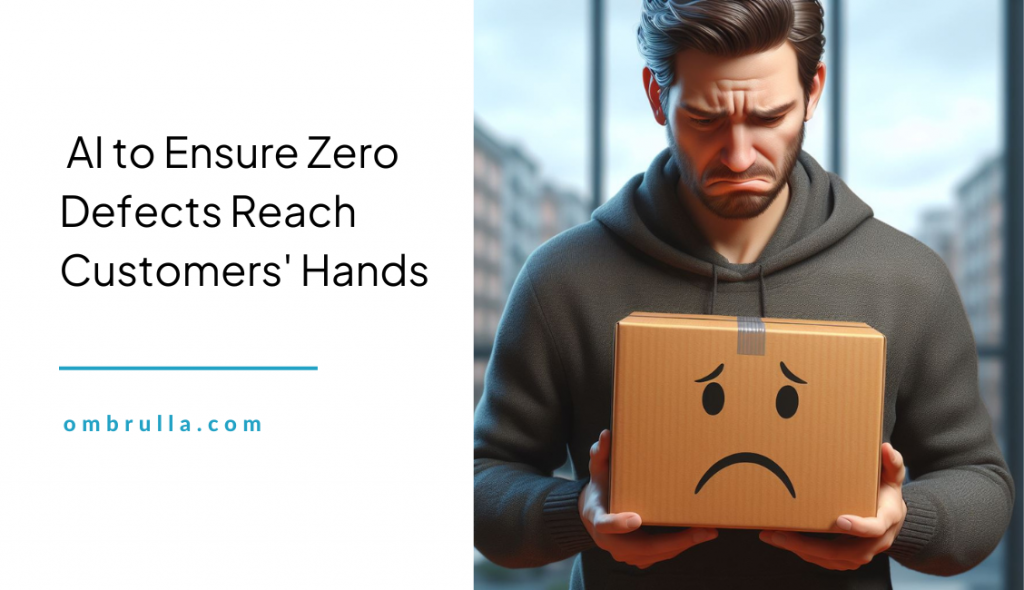
Any product manufacturer’s worst nightmare is delivering a defective item to the customer!
In today’s fiercely competitive business environment, upholding impeccable quality standards stands as an imperative across diverse sectors, spanning manufacturing, construction, and beyond. Defect inspections emerge as the vanguard against any imperfections that could compromise the safety, reliability, or performance of products. Positioned as the first line of defense, these inspections are pivotal in safeguarding customer satisfaction, preserving brand reputation, and ensuring compliance with stringent industry regulations. In essence, defect inspections constitute a foundational pillar upon which the integrity and trustworthiness of businesses are built.
In the dynamic landscape of modern industries, the significance of defect inspections transcends mere procedural necessity; it embodies a commitment to excellence and unwavering dedication to delivering products of unparalleled quality. By meticulously scrutinizing every aspect of production for flaws, faults, or irregularities, defect inspections establish a proactive approach to quality assurance, preempting potential issues before they escalate into costly liabilities. Moreover, they signify a proactive stance towards customer-centricity, signaling to consumers a steadfast commitment to delivering products that meet and exceed their expectations, thereby fostering long-term loyalty and brand advocacy.
The Role of AI in Defect Inspections
AI technologies play a pivotal role in revolutionizing defect inspection processes across various industries. One prominent application is in the steel manufacturing sector, where quality control is of paramount importance. Machine learning algorithms can be trained on extensive datasets of steel defects, ranging from surface imperfections to structural weaknesses.
Moreover, AI Visual Inspection processes offer significant advantages in terms of efficiency and cost-effectiveness. Traditional manual inspection methods are often time-consuming and prone to human error. However, with AI, the inspection process can be automated, leading to faster turnaround times and reduced labor costs.
Furthermore, AI’s capability to analyze textual data through natural language processing (NLP) algorithms complements visual inspection methods. In the steel manufacturing industry, textual data such as maintenance logs, equipment reports, and quality control documents contain valuable information that can help identify potential defect causes and preventive measures.
AI-Powered Visual Inspection and Defect Detection Systems
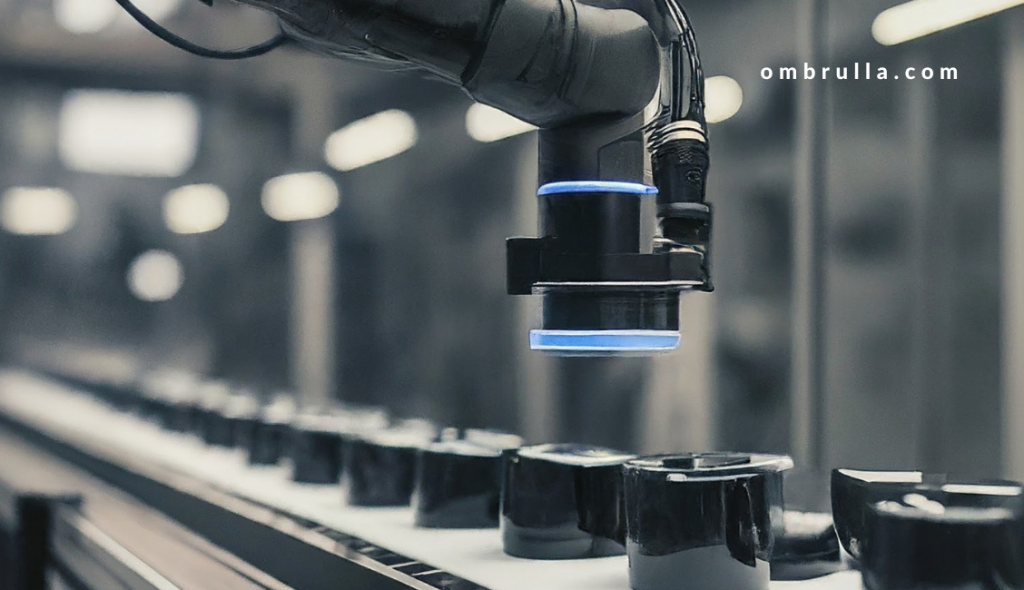
AI-Powered Defect Detection Systems merge advanced hardware and software. Cameras, sensors, and algorithms form their core. Machine learning models discern patterns from labelled datasets. They distinguish defects with precision, enhancing quality control.
In real-time inspection, these systems excel. Integrated seamlessly into workflows, they identify defects swiftly. In automotive manufacturing, AI-driven vision systems inspect car panels. Faulty components on circuit boards are identified in electronics production.
AI’s versatility shines across diverse domains. From automotive to pharmaceuticals, its applications abound. In pharmaceuticals, AI analyzes images, ensuring quality standards. These varied applications elevate defect detection’s accuracy and efficiency.
Integration of AI with Existing Inspection Processes
Integrating AI into existing defect inspection workflows involves several key steps to ensure a smooth and successful transition:
- Assessment of Current Processes: The first step is to thoroughly evaluate the existing defect inspection methods, including manual inspection processes and any automated systems in place. This assessment should identify strengths, weaknesses, and inefficiencies in the current workflow.
- Evaluation of Data Infrastructure: Industries need to assess the quality, quantity, and accessibility of data available for defect detection. This evaluation involves examining data sources, storage systems, and data management practices to determine their compatibility with AI-driven solutions.
- Skills and Resources Audit: A comprehensive audit of workforce skills and resources is essential to gauge the readiness of the organisation for AI integration. This audit should identify any skill gaps or training needs among employees who will be involved in implementing or using AI-powered inspection systems.
- Feasibility Analysis: Based on the assessment of current processes, data infrastructure, and workforce capabilities, industries can conduct a feasibility analysis to determine the potential benefits and challenges of AI adoption. This analysis should weigh factors such as cost, time, and expected outcomes.
- Collaborative Planning: Collaboration between domain experts, data scientists, and software engineers is crucial for developing a customized AI solution tailored to the specific needs of the industry. This collaborative planning phase involves defining project goals, selecting appropriate AI technologies, and designing an implementation roadmap.
- Addressing Challenges: Industries must proactively address challenges such as data quality, model interpretability, and regulatory compliance during the implementation of AI-powered inspection systems. This may involve implementing data cleaning and preprocessing techniques, developing interpretable AI models, and ensuring adherence to relevant regulations and standards.
- Transparency and Accountability: To maintain trust and credibility in defect detection processes, industries must ensure that AI algorithms are transparent, accountable, and explainable. This requires implementing mechanisms for model explicability, documentation, and auditability to provide insights into the decision-making process.
- Knowledge Transfer and Training: As AI-powered inspection systems are deployed, industries should invest in knowledge transfer and training programs to equip employees with the necessary skills to effectively use and maintain these systems. This may include providing training on AI concepts, tools, and best practices.
- Continuous Improvement: Continuous monitoring and evaluation of AI-powered defect inspection systems are essential for identifying areas of improvement and optimization. Industries should establish feedback loops and mechanisms for gathering insights from users and stakeholders to drive continuous improvement efforts.
By following these step-by-step guidelines, industries can effectively integrate AI into existing defect inspection processes, unlocking the full potential of AI-driven solutions for improved efficiency and quality control.
Benefits of AI-Driven Defect Inspections:
- Improved Accuracy and Reliability: AI-driven defect detection systems excel in accuracy and reliability over manual methods. Leveraging machine learning algorithms trained on vast datasets enhances precision. Consequently, they minimize false positives and negatives, ensuring product quality and customer satisfaction. Ultimately, this advancement enhances overall product quality and customer satisfaction.
- Reduced Inspection Time and Costs: Moreover, AI-driven inspections drastically reduce time and costs compared to manual methods. Through automation, inspection workflows are streamlined, saving labor costs and minimizing downtime. Consequently, human inspectors can focus on complex tasks, further boosting efficiency. Consequently, this efficiency translates to cost savings and optimized resource allocation.
- Facilitates Predictive Maintenance: Additionally, AI-powered defect detection enables proactive identification through predictive maintenance capabilities. By continuously monitoring, it detects early warning signs, allowing preventive measures. Consequently, this proactive approach minimizes downtime and reduces repair costs, ultimately improving equipment reliability and operational efficiency.
- Real-time Monitoring and Analysis: Furthermore, AI enables real-time monitoring and analysis, driving continuous improvement. It identifies patterns and trends for quick issue resolution. Consequently, companies can promptly implement corrective actions, preventing defects. Consequently, this approach optimizes quality control processes and enhances product quality.
Overall, the adoption of AI-driven defect detection systems offers numerous benefits for industries, including improved accuracy and reliability, reduced inspection time and costs, enhanced predictive maintenance capabilities, and real-time monitoring and analysis of defects. By harnessing the power of AI, companies can optimize quality control processes, improve product quality, and gain a competitive edge in the marketplace.
Future Outlook and Emerging Trends
The future of AI in defect inspections holds immense promise, with emerging technologies and trends poised to further advance the field. Edge AI solutions, which perform computation and decision-making locally on devices, offer opportunities for decentralized defect detection in remote or resource-constrained environments. Autonomous drones equipped with AI-powered vision systems can revolutionize inspection processes in infrastructure, agriculture, and energy sectors, enabling rapid and comprehensive defect detection across large areas. Continued research and development in AI-driven defect detection will unlock new capabilities and applications, driving innovation and efficiency in various industries.
Conclusion
In conclusion, defect inspections are essential for ensuring product quality, safety, and regulatory compliance across industries. The integration of AI technologies presents a transformative opportunity to enhance defect detection processes, offering advantages such as increased accuracy, efficiency, and scalability. By embracing AI-driven defect inspections, industries can improve quality control, reduce costs, and gain a competitive edge in the global marketplace. It is imperative for organisations to embrace AI-driven defect detection systems and leverage their potential for improved efficiency and quality control.
Related Posts:
AI Applications AI Data Analytics AI Defect Detection AI forecasting AI for Everyone AI in Automotive AI in Green Logistics AI in Logistics AI in Manufacturing AI in Pharmaseutical AI Inspection AI in Supply chain AI Quality Control AI Quality Controll AI Quality Inspection AI Video Analytics AI Visual Inspection AI Visual Inspection use cases Artificial Intelligence Computer Vision Defect Detection Future of AI Google Industrial AI Predictive maintenance Process Optimization Ship Inspection using AI
Leave a Reply