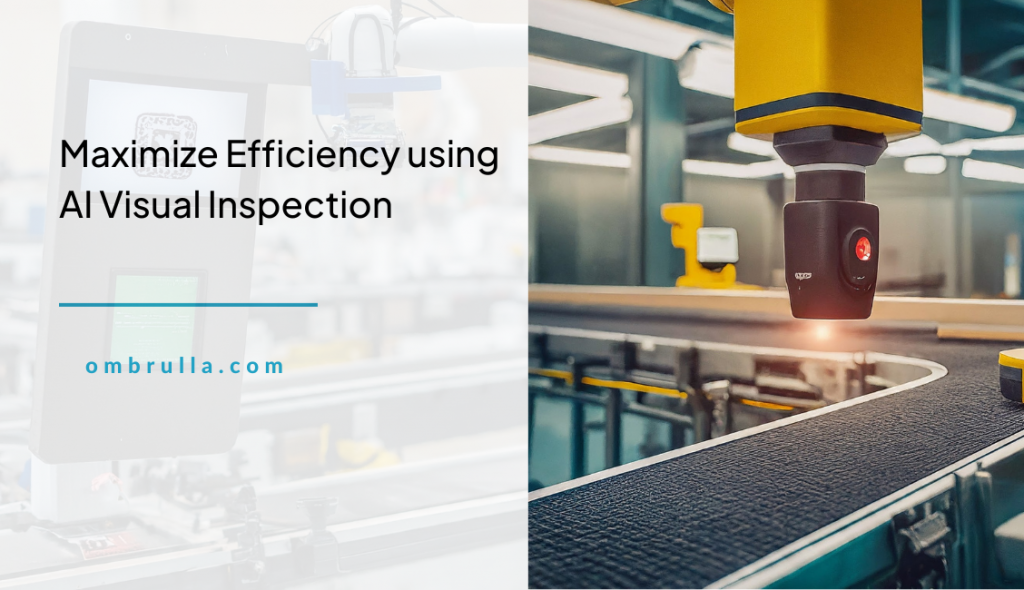
In the intricate world of manufacturing, where precision and quality are non-negotiable, ensuring the flawless production of goods is paramount. The backbone of customer satisfaction and brand reputation rests upon the ability to deliver products that meet or exceed stringent standards. Traditionally, manual visual inspection has served as the workhorse in identifying defects and upholding these uncompromising benchmarks. Human inspectors meticulously scrutinize each product, relying on their experience and expertise to detect imperfections. However, like any formidable workhorse, manual inspection comes with inherent limitations that can impede efficiency and accuracy.
Despite the dedication of human inspectors, fatigue and the inherent subjectivity of visual assessment can introduce errors and variability into the inspection process. The sheer volume of products in modern manufacturing environments further compounds these challenges, making it increasingly difficult to maintain consistent quality control. As the demand for precision continues to rise, there is a pressing need for innovative solutions that can augment or replace traditional inspection methods. Enter the era of artificial intelligence-powered visual inspection – a revolutionary alternative that has the potential to transcend the limitations of manual inspection, bringing unprecedented levels of accuracy, speed, and efficiency to the manufacturing landscape. In this blog post, we will delve into the constraints of manual inspection, explore the capabilities of AI visual inspection, and examine the transformative impact this technology can have on the manufacturing industry.
The Drawbacks of Manual Inspection
Subjectivity and Inconsistency:
Imagine a bustling production line churning out thousands of tablets every day. Each tablet passes through a team of human inspectors tasked with identifying defects on the screen. However, the human eye isn’t perfect.
- Fatigue: Inspecting a monotonous stream of products for hours can lead to eye strain and fatigue. A tired inspector might miss a subtle hairline crack on a screen, especially if the lighting isn’t ideal.
- Variations in Perception: Not all inspectors have the same level of experience or visual acuity. A seasoned inspector might be able to differentiate a tiny speck of dust from a minor scratch, while a newer inspector might mistakenly flag both as defects. This inconsistency can lead to:
- False positives: Perfectly good tablets being flagged for rework, wasting time and resources.
- False negatives: Defective tablets slipping through inspection and reaching customers, potentially harming brand reputation.
Here’s a real-world example: A car manufacturer might have inspectors checking for paint imperfections. One inspector might be more lenient towards minor swirls, while another might flag them all as defects. This inconsistency can create confusion and slow down production.
Speed Limitations:
Human inspectors can only examine products so quickly. Even the most experienced inspector can only process a limited number of items per hour. This becomes a major bottleneck as production lines ramp up to meet increasing demand.
Imagine a conveyor belt overflowing with boxes of sneakers waiting for inspection. The growing backlog disrupts production schedules and leads to delays. This can have a domino effect, impacting delivery timelines and customer satisfaction.
For instance, a toy factory might struggle to keep up with holiday demand if its inspectors can’t keep pace with the production line. This could lead to stock shortages and lost sales opportunities.
Cost and Scalability:
Maintaining a large team of inspectors is a significant financial burden. Manufacturers need to factor in:
- Salaries and Benefits: Hiring and training a sufficient number of inspectors can be expensive, especially for highly skilled positions requiring specific expertise.
- Overhead Costs: Providing inspectors with proper equipment, workspace, and breaks adds to the overall cost.
Scaling up a manual inspection team can be challenging. Finding qualified inspectors takes time and resources, and integrating them into existing workflows requires additional training. This inflexibility makes it difficult for manufacturers to adapt to fluctuating production demands.
For example, a clothing manufacturer might experience a surge in orders during a seasonal sale. However, expanding the inspection team quickly to handle the increased workload might not be feasible, potentially leading to missed deadlines.
Safety Concerns:
Certain manufacturing environments present inherent hazards to human inspectors. Consider these examples:
- Exposure to Fumes and Chemicals: Inspecting freshly welded car parts might expose inspectors to heat and harmful fumes. Similarly, inspecting chemical containers could lead to risks of skin or respiratory problems.
- Repetitive Strain Injuries: Manufacturing lines often involve repetitive motions. Inspectors constantly handling products for long periods are susceptible to repetitive strain injuries like carpal tunnel syndrome.
AI visual inspection eliminates these safety risks. Machines can handle inspections in hazardous environments, keeping human workers safe. This not only protects employees’ well-being but also reduces the risk of accidents and associated costs.
Introducing AI Visual Inspection:
At the heart of AI-powered visual inspection lie cutting-edge technologies, prominently among them being computer vision and deep learning. Computer vision endows machines with the ability to “see” and interpret visual information, enabling them to process and understand the nuances of images. Simultaneously, deep learning facilitates the evolution and refinement of artificial intelligence systems through continuous exposure to vast datasets. This dynamic combination allows AI systems to develop a sophisticated understanding of visual elements, paving the way for more advanced and nuanced analyses.
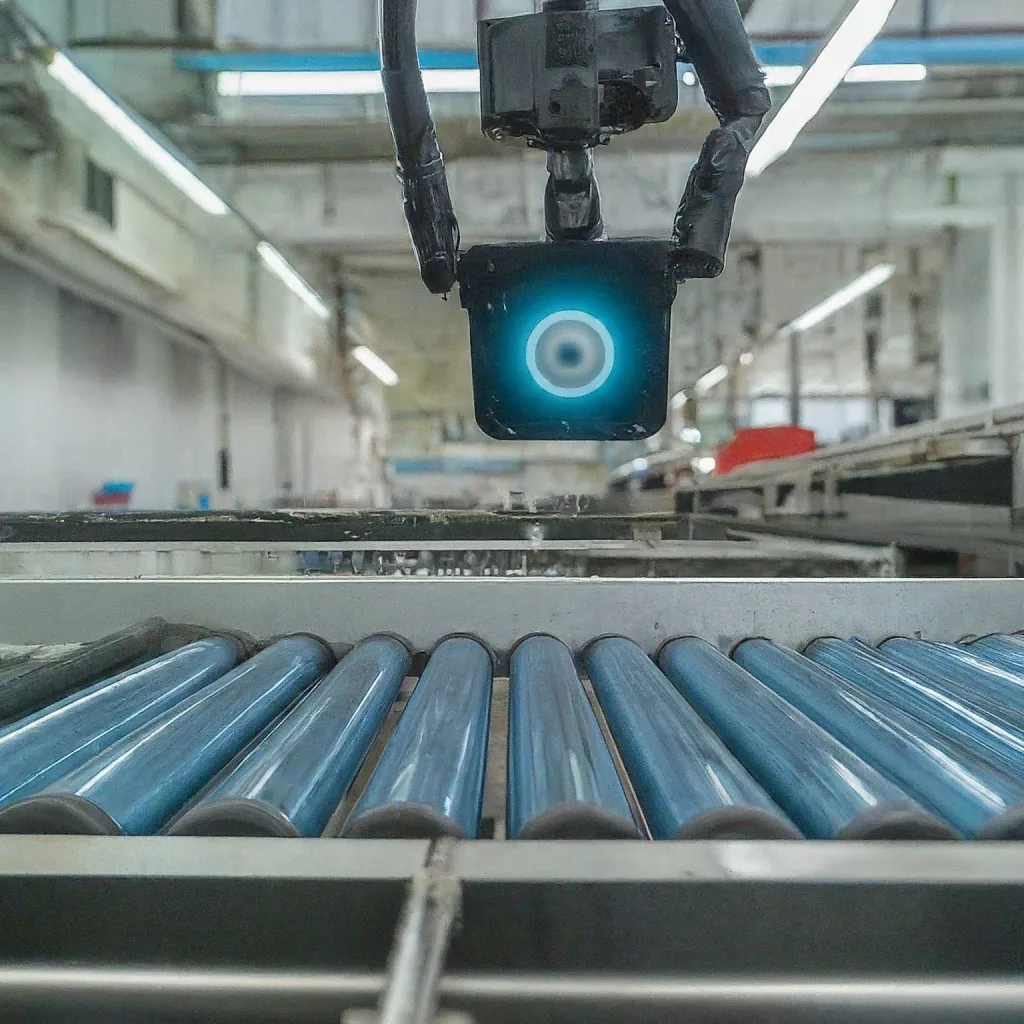
Consider a scenario where AI visual inspection is applied to the manufacturing of drill bits. The system is meticulously trained on an extensive dataset comprising images of both flawless and defective drill bits. Through this comprehensive exposure, the AI algorithm discerns the intricate details that characterize a perfectly formed drill bit, encompassing factors such as shape, sharpness, and the absence of imperfections. This knowledge becomes the bedrock upon which the AI system can rapidly process incoming drill bits, swiftly identifying and flagging any deviations from the established norm.
The strength of AI-powered visual inspection lies in its ability to surpass human limitations. Unlike manual inspection, AI systems do not succumb to fatigue, ensuring consistent and tireless scrutiny of each product. Moreover, the AI’s capacity for continuous learning means that its defect identification skills improve over time, adapting to new patterns and variations. This transformative technology not only enhances the efficiency of quality control processes but also contributes to the overall advancement of manufacturing standards. In the subsequent sections of this blog post, we will delve deeper into the mechanics of AI visual inspection, exploring its applications, benefits, and potential challenges in revolutionizing quality assurance in manufacturing.
The Advantages of AI Visual Inspection:
- Objectivity and Consistency: AI systems are not susceptible to fatigue, distraction, or individual interpretations. They evaluate products based on clearly defined parameters, ensuring consistent and reliable defect detection. This consistency translates to fewer errors and a higher quality output.
- Increased Speed and Throughput: AI systems can analyze products at a much faster rate than human inspectors. This translates to a significant boost in production throughput, keeping pace with even the most demanding manufacturing lines. Imagine the smartphone production line mentioned earlier – an AI system could analyze phones in a fraction of the time it takes a human inspector, eliminating bottlenecks and keeping production flowing smoothly.
- Reduced Costs: By automating the inspection process, AI reduces the need for a large manual workforce. This translates to cost savings on salaries, training, and benefits. Additionally, AI’s ability to catch defects early in the process minimizes rework, further reducing production costs.
- Improved Safety: AI systems can handle inspections in environments that are hazardous to human health. This eliminates the risk of exposure to fumes, chemicals, or other dangers often present on factory floors.
- Data-Driven Insights: AI systems not only identify defects but also generate valuable data. This data can be analyzed to identify trends, pinpoint recurring issues in the manufacturing process, and ultimately lead to process improvements. For instance, the AI system inspecting drill bits might identify a particular drill press as consistently producing bits with minor imperfections. This data can then be used to troubleshoot and fix the drill press, preventing future defects.
Implementation Considerations:
While AI visual inspection offers numerous benefits, transitioning to this technology isn’t without its hurdles. Here’s a closer look at two key challenges manufacturers might face:
1. Data Collection and Diversity:
Training effective AI models relies heavily on data. Imagine a massive library of images – defect-free products on one side and products with various imperfections on the other. The more data the AI consumes, the better it becomes at recognizing patterns and identifying defects. However, collecting sufficient and diverse data can be a challenge:
- Quantity: Training a robust AI model requires a vast amount of data. This might necessitate capturing images of thousands, or even millions, of products, encompassing a wide range of variations.
- Example: A clothing manufacturer implementing AI for inspecting shirts might need a dataset containing images of shirts with various types of tears, stains, and stitching imperfections. This ensures the AI can effectively identify these defects across different colors, fabrics, and sizes.
- Diversity: The data used to train the AI model needs to be diverse and representative of real-world scenarios. This includes capturing images under different lighting conditions, at various angles, and with different types of background noise.
- Example: An AI system inspecting car parts on a factory floor needs to be trained on images that account for variations in lighting – bright overhead lights, shadows cast by machinery, etc. Failing to account for these variations could lead to the AI misidentifying defects in certain lighting conditions.
2. Integration with Existing Infrastructure:
Integrating AI systems seamlessly into existing manufacturing processes can be complex. Here’s why:
- Compatibility: Manufacturers might have existing equipment and software that may not be readily compatible with the AI system. This can necessitate upgrades or modifications to ensure smooth data flow and communication between the AI and the production line.
- Example: A factory with an outdated conveyor belt system might need to implement upgrades to handle the faster processing speed of AI inspection compared to manual inspection.
- Workflow Adjustments: The introduction of AI might require adjustments to existing workflows. Inspectors used to manually examining products might need to be retrained to handle data analysis, system troubleshooting, or quality control tasks that complement the AI system’s capabilities.
However, there’s good news! As AI technology matures, these challenges are becoming less daunting:
- Pre-trained Models: Many AI vendors offer pre-trained models for specific industries or applications. These models can serve as a starting point, reducing the amount of data a manufacturer needs to collect themselves.
- Data Annotation Tools: Advanced software tools can streamline the data annotation process, which involves labeling images to identify defects. These tools can automate repetitive tasks and improve data labeling accuracy.
- Modular AI Systems: Modern AI solutions are designed with greater modularity, allowing for easier integration with existing infrastructure. Additionally, many vendors offer support services to help manufacturers navigate the integration process.
By understanding these challenges and leveraging the evolving capabilities of AI technology, manufacturers can position themselves to overcome these hurdles and unlock the full potential of AI visual inspection.
The Future of Quality Control:
The role of AI in manufacturing is expanding beyond visual inspection. Predictive maintenance, where AI predicts when equipment is likely to fail, and self-optimizing processes are becoming integral parts of the manufacturing landscape. Embracing AI is crucial for building a more efficient, reliable, and forward-looking future in manufacturing.
In conclusion, the integration of AI visual inspection is not just a leap forward in quality control but a transformative shift towards a smarter and more advanced manufacturing landscape. Manufacturers who embrace this change stand to benefit from improved efficiency, reduced costs, and a competitive edge in an increasingly sophisticated market.
AI Applications AI Data Analytics AI Defect Detection AI forecasting AI for Everyone AI in Automotive AI in Green Logistics AI in Logistics AI in Manufacturing AI in Pharmaseutical AI Inspection AI in Supply chain AI Quality Control AI Quality Controll AI Quality Inspection AI Video Analytics AI Visual Inspection AI Visual Inspection use cases Artificial Intelligence asset health monitoring asset performance management asset performance management software asset tracking Computer Vision Defect Detection equipment tracking Future of AI Google Industrial AI iot asset management Predictive maintenance predictive maintenance systems Process Optimization Ship Inspection using AI
Leave a Reply